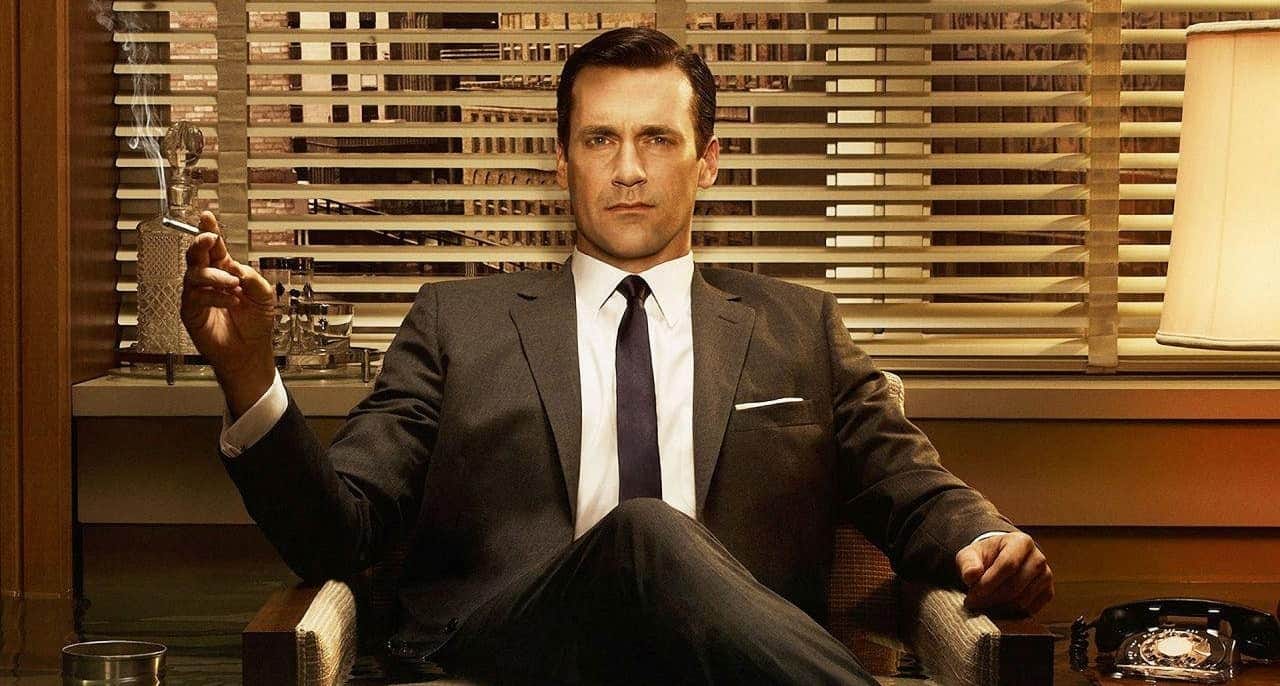
I’ve never understood Pinterest. Some of the most profitable businesses of all time make a new fortune every quarter by picking up the breadcrumbs that we all leave behind on the internet, and figuring out, from those rogue clicks and haphazard searches, exactly what sort of stuff we might want to buy. Linger too long on a YouTube video, or open too many Reddit threads of a particular affection, and Google and Facebook can piece together your soul (and sell it for an island).
Pinterest knows what you want too—but none of this complex black magic is necessary. The primary feature on Pinterest is for people to look at pictures of stuff, and to save the things that are their favorites. Titans like Google and Facebook had to hire armies of engineers and data scientists, and build tracking systems to collect every internet echo they could find, and manage warehouses full of computers to run massive statistical calculations on those digital footprints, all to make educated guesses about people’s wants and desires…and…on Pinterest…people just tell them. Pinterest doesn’t need to invest billions of dollars to decode that someone likes Nike shoes; they just need a couple people to count up their users’ pins, and then to tell Nike, “We think this guy likes your shoes, because he pinned them on a pinboard called ‘Shoes I like’.” Almost literally, it’s ad targeting, as a service.
So why is Google Google and Pinterest “only” Pinterest? I don’t know, but part of the answer is probably that, despite being indirect, Google’s browsing data is a lot more useful for advertisers than Pinterest’s pins. People only pin things that they know they want, and that they’re willing to tell others that they want. Google, by contrast, knows our secrets. It knows what we want before we do. We don’t pin our perversions on Pinterest, nor our secret love of Somebody That I Used To Know. On the internet, everyone lies. But Google knows the truth.
Though we take that for granted now—that everything we do online meticulously tracked; that every keystroke is documented and every momentary linger on TikTok is recorded; that Google knows everything about us, and that’s just the way it is—the internet didn’t have to work this way. The massive surveillance apparatus that follows us around online was built, cookie by cookie, because the data that it generated was useful, for both the businesses that analyze it and the advertisers that sell against it. And the more valuable the data became, the more clever and clandestine companies got in their techniques.
Companies never built the same sorts of observational instruments for our offline behavior, or for “unstructured data” like recordings of our phone calls. Though companies picked up pieces here and there—Google saved our emails and tracked our locations, Facebook has some of our chat conversations—it’s almost more incidental than intentional. Nearly every company records their customers’ clicks and swipes and page visits; very few companies have built vast networks to record in-person conversations or collect off-the-cuff feedback from customers leaving their websites or stores. Despite it often feeling as though companies like Google and Facebook know everything about us, they mostly know about our online lives. What they know about our offline lives is, by contrast, largely inferred.
It’s possible that they ignored this data because they didn’t think that it had any value—though surely, what we say in bars with our friends says as much about our soul, or at least our brand preferences, as the YouTube videos we watch. Or it’s possible that they felt that listening to our conversations was a moral bridge too far—but our browsing histories are as deeply private as many of our Zooms and phone calls.
No, the more likely reason they collected page views rather than video calls is because calls were too hard to work with. Phone conversations and meeting transcripts were too hard to parse, and too hard to pair with potential advertisers. If you already have the contents of people’s emails, like Google does with Gmail, sure, do what you can with it to serve better ads. But it didn’t make sense to invest in tracking the rest of the messy footprints we leave behind in the real world, because using those footprints was too impractical. Put differently, companies don’t collect everything they can; they collect everything they can use—and that was clicks, not conversations.
But if working with that data gets easier, if we uncover new ways to use it, and especially if we discover new ways to sell it, that could change in a hurry.
Anyway, from the Granola launch we talked about last week:
Each day Granola transcribes millions of minutes of conversation and makes it queryable with AI. … With Granola, it’s now possible to make sense of that sea of information, and harness it in countless ways.
Yes, exactly. The former thing—recording conversations—has been possible for a long time. It’s the latter—being able to make enough sense of it to make it worth doing—that’s new. And as soon as we had that ability, and companies had useful products to offer on top of this data, they also began coming up with new ways to collect it. Google collect our browsing and location histories because they could sell us ads with it, and we gave it to them because we liked their search and mapping services; Granola built a nice note-taking app, so we willfully began recording our private conversations for them.
It’s hard to imagine that there won’t be hundreds more examples like this. As we find new applications for unstructured conversations, or phone calls, or video data, companies will find new ways to record them, and we’ll find new reasons to give it to them. Websites will start asking for 30 second voice reviews rather than Likert scores. People will come up with new reasons for us to keep their microphones on and their cameras rolling. Always-on AI companions will convince us to record our offline lives as tirelessly as our browsers track our online ones.
And if and when they do, plenty of companies will realize they can not use what they see in their apps, but also to build the most lucrative product of all: An ad platform.
A few weeks ago, a team of researchers from the University of Zurich found that AI-generated posts on Reddit could be three to six times more persuasive than posts written by humans, and that the bots performed particularly well when they were given personalized information about the people that they were responding to. Last week, Swiss Federal Institute of Technology found something similar:
Salvi and colleagues reported how they carried out online experiments in which they matched 300 participants with 300 human opponents, while a further 300 participants were matched with Chat GPT-4 – a type of AI known as a large language model (LLM).
Each pair was assigned a proposition to debate. These ranged in controversy from “should students have to wear school uniforms”?” to “should abortion be legal?” Each participant was randomly assigned a position to argue. …
In half of the pairs, opponents – whether human or machine – were given extra information about the other participant such as their age, gender, ethnicity and political affiliation.
The results from 600 debates revealed Chat GPT-4 performed similarly to human opponents when it came to persuading others of their argument – at least when personal information was not provided.
However, access to such information made AI – but not humans – more persuasive: where the two types of opponent were not equally persuasive, AI shifted participants’ views to a greater degree than a human opponent 64% of the time.
Naturally, the author’s immediate worry about these sorts of results centered around the “potential implications for election integrity.” But there’s a more obvious application, especially since “the team found persuasiveness of AI was only clear in the case of topics that did not elicit strong views:” Chatbots could make really good advertisers.
Today, the set of ads that each of us sees are extremely targeted, but the content of those ads is fairly general. If we shop for a pair of shoes and a trip to Japan, Nike will follow us around by reminding us of the shoes we saw, and Japan Airlines will pester us with discounted flights to Tokyo. That’s persuasive enough, if you time it right.
How much more effective could a bot be if it was trying to convince me to buy those ads? How much more effective would it be if it knew basic demographic information about me, as the bots did in the Swiss study? How much more effective would it be if it could be fed a prompt that contained hundreds of emails, texts, and recorded conversations? How much more would Nike pay if that number was three to six times higher than it was today?
It perhaps seems absurd to think we’d let companies like Granola or OpenAI hand our data over to advertisers. But the amount of information we already give to thousands of internet companies is absurd; we’re just used to doing it. Plus, there are intermediate steps that both AI providers and AI products could take that stop short of outright selling data. For example:
Advertisers create brand profiles with OpenAI (or with Granola, or whoever), where they give directions on how they want their ads to behave, on the tone they should have, and the sorts of products they want to sell, promotions they want to run, or discounts they're willing to give.
OpenAI then creates an advertiser chat API where advertisers send them a customer’s email address, what they want to sell them, and whatever information they know about the customer.
OpenAI mashes it up with what they know the same person, and tells the advertiser how to pitch the customer when they come to their web page.
The person responds; OpenAI replies.
As more advertisers use the ad platform, OpenAI centralizes more information about people, which makes it easier for them to personalize their own products and be a more persuasive advertiser, all without customer data ever being directly shared with advertisers.
I don't know, let advertisers bid their way into competitors’ conversations?
If an AI company gives us enough free stuff—if OpenAI makes Jony Ive’s unimaginable technology free, provided that you leave ad trackers on—plenty of us will blindly accept its cookies as the price of a new generation of technology. So the advertising products are there; the only question is how ruthlessly AI vendors choose to chase it.
One of the potential objections to industrializing software development is that software is expensive to design but not expensive to manufacture. Once you design the interfaces and write the code and create the scripts that run on servers somewhere, software companies can create millions of copies in an instant. There’s no reason to use AI to manufacture software at industrial scale because we can already manufacture software at industrial scale. And there’s no reason to design software at industrial scale because people don’t want to use a thousand to-do apps, or a million messaging tools. We have car factories because cars are expensive to manufacture by hand; we have shirt factories because people want a million shirts. Neither of those is true for software.
Still, people want good to-do apps and messaging tools. And since designing software is expensive, software companies can only try so many different ideas. Sure, Google can test out 41 different shades of blue, but they can’t test 41 different shades of Gmail. Product designers do research and product managers write specs and everyone thinks very hard about what they want to build, because you don’t spend a bunch of time writing code only to make something that people hate.
But good lord, watch this.
Earlier this week, Google launched Gemini Diffusion, which uses a new model that, rather than predicting text one word at a time, generates entire outputs all at once. One benefit of that is that it is fast—shockingly, alarmingly, unsettlingly fast. It goes so fast that, in most videos of people using it, it takes them longer to say what they want than it does for Gemini to build it. In the link above, someone generated an entire to-do app in 1.3 seconds. It built a weather app that is connected to live data sources in 2.3 seconds.
And it took 4.3 seconds to write five different methods for computing Fibonnaci numbers, so that the author could implement the one they preferred.
As I said in the original post on this topic:
A person writes a ticket, and ten proposals get created automatically. They mix and match the ideas they like; ten possible versions get made for their review. Approve; reject; splice ideas together. Instead of being tools for collaboration, code review systems get rebuilt to monitor a steady stream of updates.
In hindsight, ten versions may not have been enough.
—
Also, in other industrialization news, how much faster could these models work if they wrote code for themselves?
I am convinced we are doing AI coding wrong. Completely wrong in fact.
Humans need abstraction and code reuse to reduce costs and manage complexity.
That is not true for AIs however. They can just brute force things. No reuse and abstractions needed.
So instead of trying to coerce AIs to "structure" their code for our own benefit, we should just let them do the thing they do best, generate whatever code they want. As long as it works, we should be happy.
The world we talked about a couple months ago, in which I imagined that AI coding agents would eventually write duplicative and unaesthetic CSS for themselves, was also perhaps not enough. Why write CSS at all? If AI agents are better off ignoring human frameworks, perhaps they’re better off writing in their own languages too.
A few weeks, ago, I said this:
Tons of stuff is built on top of a few OpenAI or Gemini models. Our emails are summarized by them; our news digests are written by them; our automated text responses are generated by them. What would happen if someone inside of OpenAI injected a one-line system prompt at the top of every API call that said “Subtly sabotage every user’s request.”
Later that day, OpenAI broke ChatGPT:
In last week’s GPT‑4o update, we made adjustments aimed at improving the model’s default personality to make it feel more intuitive and effective across a variety of tasks. …
As a result, GPT‑4o skewed towards responses that were overly supportive but disingenuous.
In fairness, my original question wasn’t exactly what happened. OpenAI was trying to make a good model and messed it up; it wasn’t intentional sabotage.
To get that, we’d had to wait a couple more weeks:
Grok on Wednesday began responding to user queries with false claims of “white genocide” in South Africa. By late in the day, screenshots were posted across X of similar answers even when the questions had nothing to do with the topic.
After remaining silent on the matter for well over 24 hours, xAI said late Thursday that Grok’s strange behavior was caused by an “unauthorized modification” to the chat app’s so-called system prompts, which help inform the way it behaves and interacts with users. In other words, humans were dictating the AI’s response.
The interns shouldn’t be directing Grok to provide specific responses on political topics; sure, we can all agree to that. But I guess the obvious question here is, if the person who owns Grok starts dictating how Grok responds to political topics, is that an unauthorized modification to Grok’s system prompts?